ВУЗ: Не указан
Категория: Не указан
Дисциплина: Не указана
Добавлен: 09.12.2023
Просмотров: 14
Скачиваний: 1
ВНИМАНИЕ! Если данный файл нарушает Ваши авторские права, то обязательно сообщите нам.


DOI: 10.1049/cim2.12069



ORIGINAL RESEARCH
A taxonomy of factors influencing worker's performance in human–robot collaboration

Department of Industrial Engineering, University of Salerno, Fisciano, SA, Italy
Correspondence
Valentina Di Pasquale, Department of Industrial Engineering, University of Salerno, Via Giovanni Paolo II, 132, 84084 Fisciano, SA, Italy.
Email: vdipasquale@unisa.it
Funding information
Ministero dell'Università e della Ricerca, Grant/ Award Number: Project PRIN – 2017FW8BB4
Abstract
The occurrence of human errors significantly affects the performance and economic results of production systems. In this context, Human Reliability Analysis (HRA) methods play a key role in assessing the reliability of a man–machine system. Several HRA methods use Performance‐Shaping Factors (PSFs), that is, all the aspects of human behaviour and environment that can affect human performance, to evaluate the Human
Error Probability (HEP). However, despite the greater emphasis given by researchers to define of PSFs in recent years, the changes caused by the new enabling technologies implemented in manufacturing systems and derived from the Industry 4.0 paradigm have not yet been fully explored. Focussing on Human–Robot Collaboration (HRC) in pro- duction systems, the authors aim to define a PSF taxonomy that is useful for HEP evaluations in collaborative environments. To the best of the authors' knowledge, HRA approaches have not been investigated yet for HRC applications. The proposed taxon- omy, which results from the integration of the most significant factors impacting workers' performance in HRC into the PSFs provided by an HRA method, can represent an important contribution for researchers and practitioners towards improving HRA methods and their applications in the context of Industry 4.0.
KEYW ORDS
cobot, collaborative robots, human factors, human performance, human reliability analysis, Operator 4.0, PSF
-
| INTRODUCTION
One of the main purposes of Industry 4.0 is to overtake traditional manufacturing systems by fully integrating physical and virtual worlds and creating systems in which everything is connected but without replacing humans [1]. For this reason, the development of good relationships between humans and technologies has become increasingly important. It is nowadays
essential to establish trusting and interaction‐based relation- ships aimed at empowering operators with new skills and
enhancing their physical, perceptual and cognitive abilities through the implementation of so‐called enabling technologies.
traditional industrial robots can achieve high levels of accuracy, speed and repeatability in performing the tasks they are pro- gramed for, but they lack versatility. In contrast, human workers have innate flexibility and can adapt to unforeseen events and dynamic and complex working environments [2]. With collaborative robotics, the advantages of traditional automation are combined with the flexibility and cognitive and soft skills of human workers.
Generally, all forms of interactions between humans and robots are defined as Human–Robot Interaction (HRI). Ac- cording to the characteristics of workspace, working time, aim and contact, it is possible to distinguish three main types of
-- -
-





labelled as a Human–Robot Coexistence (HRCoex). In this case, both entities must not necessarily have the same aim because they can operate on very different tasks.
-
In contrast, interaction is defined as Human–Robot Coop-
eration (HRCoop), which is when humans and robots are working on the very same aim and fulfiling the requirements of time and space (as in HRCoex) at the same time.
-
Finally, Human–Robot Collaboration (HRC) can be defined
as a particular state in which a robot system and an operator work on simultaneous tasks within a collaborative environ- ment characterised by no temporal or spatial separation of the robotic and human activities. Typically, this requires a coordinated, synchronous activity from all parties where direct physical contact (haptic or auditory) is also allowed.
With the introduction of HRC in the manufacturing context, working conditions have radically changed with the ability of humans and cobots to move simultaneously. More- over, workers must get used to sharing a common space with the cobot and without real physical separations. For this
reason, to ensure a good level of collaboration, the psycho‐ physical state of the human involved in operations with the
robot must be taken into account [4]. The success of the collaboration, and consequently, the performance of the task are significantly affected by the emotions and cognitive process of the people involved in this new type of close interaction [4]. Результаты недавнего обзора литературы [5] показали, что с точки зрения человека СЧР в основном влияет на безопасность, эргономику и производительность. Исследование подтвердило положительное влияние ВЧР на производительность операторов, несмотря на то, что было показано, что внедрение совместной роботизации на заводе также может негативно повлиять на поведение людей и производительность некоторых работников. Пробел, выявленный в результате обзора, заключается в том, что человеческий фактор, который следует учитывать при оценке производительности оператора, еще не исследовался в отношении внедрения СЧР в производственном контексте. Этот недостаток в литературе становится еще более очевидным, когда уделяется внимание методам анализа человеческого фактора. Методы количественного анализа человеческого фактора (АВЧФ) направлены на количественную оценку вероятности человеческой ошибки в зависимости от выполняемой оператором деятельности и факторов окружающей среды, индивидуальных и организационных факторов, которые могут улучшить или ухудшить работу человека, которые также называются Факторы‐ определяющие производительность (ФОП). В настоящее время не существует стандартного набора ФОП, используемого среди методов АВЧФ, которые включают отношения между людьми и сотрудничающими роботами. Из-за особенностей этих технологий, внедрение которых вызвало проблемы, не представляется возможным сразу определить общую структуру для АВЧФ на основе общего набора ФОП. По этим причинам, учитывая полученные результаты и пробелы, отмеченные в [5], настоящая статья посвящена определению предварительной таксономии ФОП в задачах совместной работы человека и робота для оценки влияния АВЧФ на СЧР. В отличие от нынешнего подхода к оценке АВЧФ, который не включает аспекты, связанные с внедрением стимулирующих технологий, основной целью настоящей работы является первичное расследование этого случая.
Текущая статья организована следующим образом: В разделе 2 представлен обзор методов АВЧФ. Используемая методология исследования описана в разделе 3, а в разделе 4 представлены основные результаты, полученные в соответствии с методологией исследования, и предлагаемая таксономия, полученная в результате анализа. Наконец, в разделах 5 и 6 представлены основные обсуждения и выводы исследования, а также возможные направления будущих исследований
-
| ОСНОВЫ АНАЛИЗА ЧЕЛОВЕЧЕСКОГО ФАКТОРА
При оценке надежности системы необходимо принимать во внимание все ее компоненты, включая человеческую составляющую, которая может влиять на ее производительность даже в большей степени, чем технологическая [6]. Было подсчитано, что человеческие ошибки являются причиной 60-80% несчастных случаев, в то время как только оставшийся процент приходится на технические сбои [6]. Таким образом, основным следствием человеческой ошибки в промышленной системе является возникновение случайного события, но незначительные ошибки могут привести к снижению производительности операции с точки зрения производительности и эффективности [7]. Дополнением к человеческой ошибке является человеческий фактор, которую можно определить как вероятность того, что человек выполнит в соответствии с требованиями задачи в течение определенного времени и не будет выполнять никаких посторонних действий, которые могут привести к ухудшению работы системы; он также определяется как способность человека выполнять выполнить заданную задачу при заданных условиях за заданный интервал времени в допустимых пределах [7, 8].
Из-за распространенности человеческих ошибок и их огромных и часто дорогостоящих последствий это исследование становится все более важной исследовательской задачей и важным направлением в рамках АВЧФ, который превратился в четко определенную дисциплину. АВЧФ играет важную роль в оценке общей надежности анализа человеко–машинной системы, и это важно для выявления причин и источников человеческих ошибок и оценки вероятности таких ошибок. Другими словами, АВЧФ можно определить как любой метод, с помощью которого оценивается человеческий фактор. Суэйн и Гуттманн [9] определили цели HRA, которые все еще актуальны, следующим образом: "Задачей АВЧФ является оценка вклада оператора в надежность системы. Точнее, цель состоит в том, чтобы спрогнозировать частоту человеческих ошибок и оценить деградацию человеко–машинных систем, которая может быть вызвана человеческими ошибками в связи с функционированием оборудования, эксплуатационными процедурами и практиками, влияющими на поведение системы’. Как правило, АВЧФ включает в себя три этапа (рис. 1), начиная от выявления источников ошибок и моделирования этих ошибок в рамках системного анализа, включая аппаратные сбои, и заканчивая количественной оценкой вероятности человеческой ошибки (ВЧО). На протяжении качественного и количественного этапов АВЧФ многие методы АВЧФ используют ФОП, то есть все экологические и поведенческие факторы, которые влияют на производительность человека и должны быть приняты во внимание [7].
В традиционном процессе АВЧФ анализ задач используется для понимания взаимодействия между людьми и системами, в результате чего выявляется соответствующая таксономия ошибок. Аналитики сначала определяют события связанные с человеческими ошибками (СЧО), то есть единицы анализа в АВЧФ. СЧО, которые можно определить как человеческие ошибки, оказывающие неблагоприятное воздействие на систему, анализируются качественно и количественно, прежде чем присваивать событиям относительные номинальные значения ВЧО. Номинальный ВЧО рассчитывается на основе деятельности оператора, и для получения количественной оценки ВЧО используются ФОП. ФОП могут действовать как мультипликаторы номинального ВЧО: когда ФОП дает положительный эффект, номинальный ВЧО уменьшается; в обратном случае он действует как увеличивающий множитель (рис. 2) [10]. ФОП определяется индивидуальными характеристиками человека, окружающей среды, организации или вида деятельности, которые повышают или снижают производительность человека и увеличивают или уменьшают вероятность человеческих ошибок [7]. Подводя итог, можно сказать, что номинальный ВЧО определяется как функция выполняемой задачи, в то время как значения ФОП зависят от человеческого фактора, организационных факторов и экологических аспектов.
Методы или подходы АВЧФ можно разделить на три категории: первое, второе и третье поколения. В таблице 1 показано распределение методов АВЧФ по годам, основные особенности трех поколений и основные подходы, разработанные исследователями.
-
| METHODOLOGICAL APPROACH
The present study analyses two different topics (‘Human Robot Collaboration’ and ‘Human Reliability Analysis’) that have been investigated individually by following different methodological approaches, and the results have been then combined to define a PSF taxonomy for collaborative tasks. The methodology approach was made up of three logical steps as shown in Figure 3.
Step 1 is based on a literature review that investigated how the operator's performance changes with HRC introduction in the manufacturing system, the results of which are thoroughly reported in [5]. Through a scoping review approach [16], the evidence was synthesised, here with the main aim of identifying

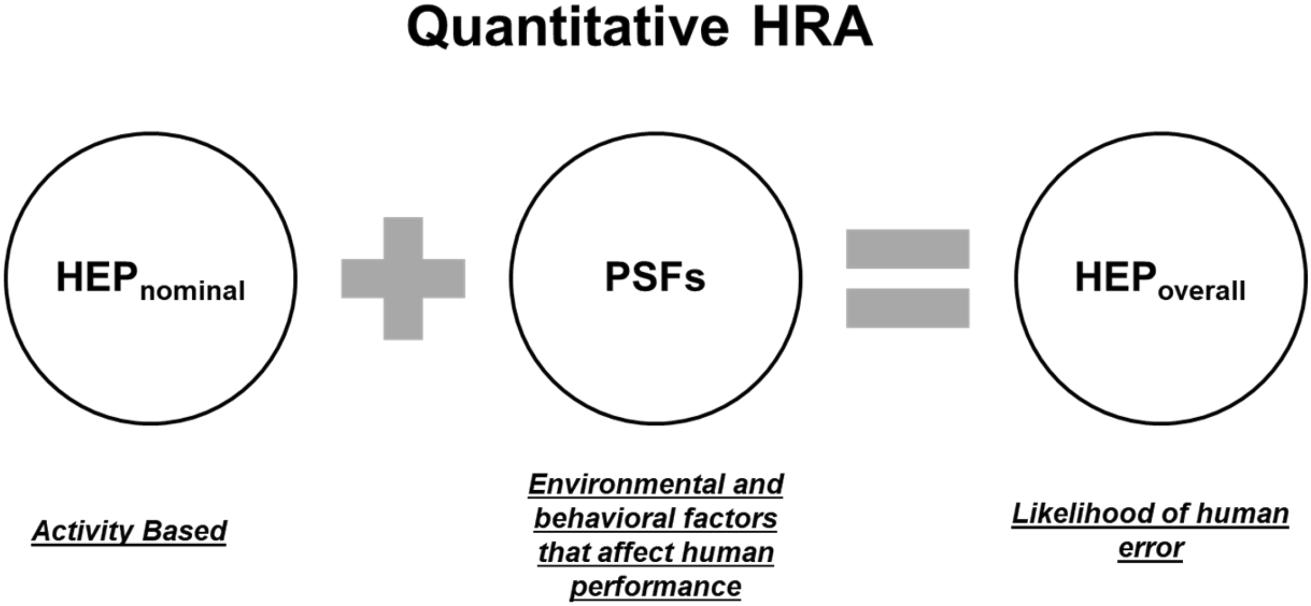
FIGURE 2 Quantitative Human Reliability Analysis (HRA) methods: nominal Human Error Probability (HEP), Performance‐Shaping Factors (PSFs) and overall HEP.
TABLE 1 Human Reliability Analysis (HRA) methodologies
Generation

First 1970–1990 Human actions are considered in a binary way, that is, as the
success or failure in obtaining the required outcome of an activity, without paying much attention to the causes and reasons of the human behaviour
Second 1990–2005 These methods focus on human performance factors and
cognitive processes considering causes of errors rather than their frequency, factor interactions that increase the probability of error and interdependencies among PSFs
Third 2005–today These methods focus on the relationships and dependencies among PSFs
Technique for Human Error Rate Prediction (THERP) [11], Accident Sequence Evaluation Programme (ASEP) [12], Human Cognitive Reliability Model (HCR) [13], Human Error Assessment and Reduction Technique (HEART) [11]
Cognitive Reliability and Error Analysis Method (CREAM) [13], Standardised Plant Analysis Risk – Human (SPAR‐H) [14]
Nuclear Action Reliability Assessment (NARA) [15]

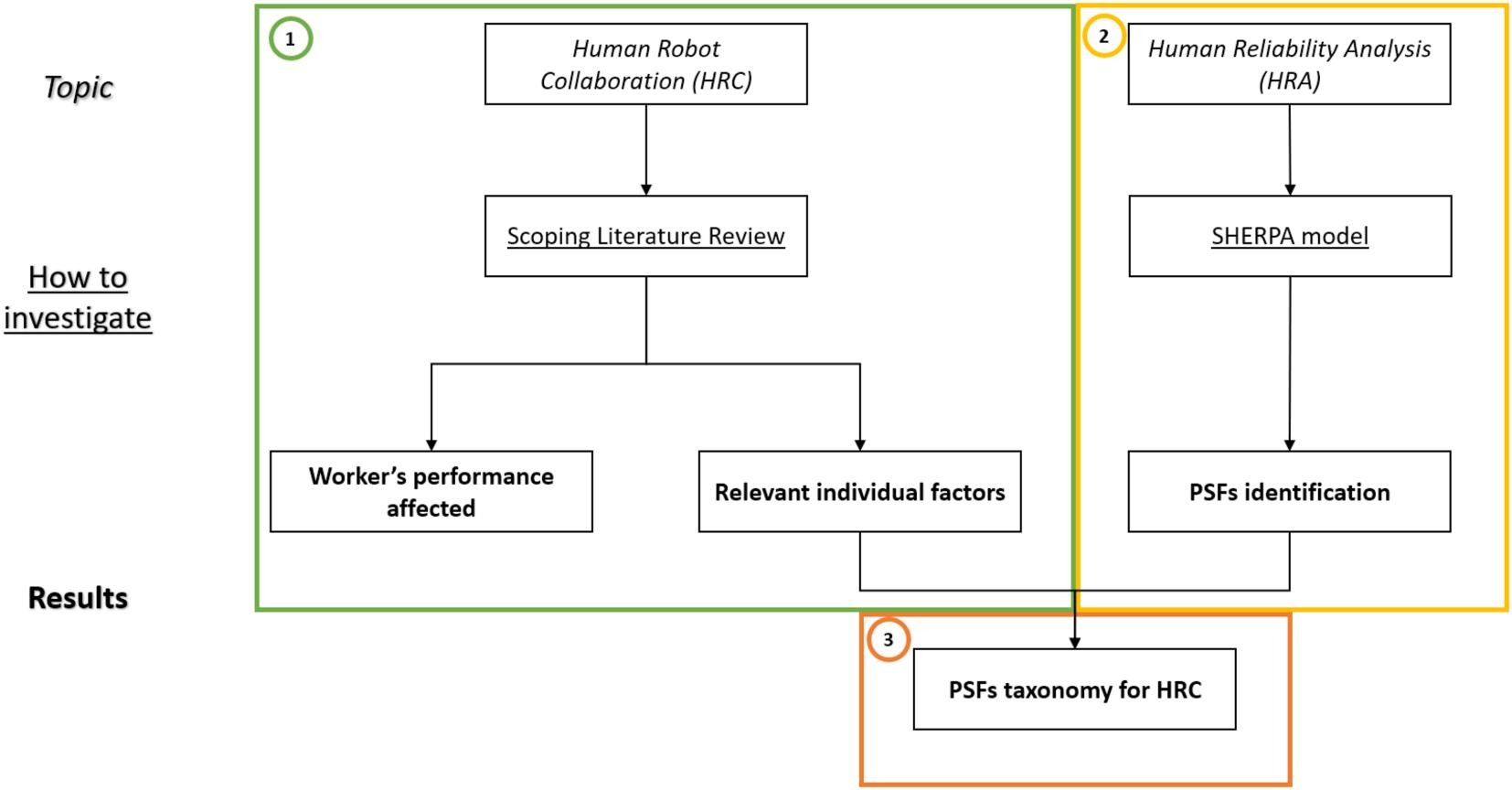
FIGURE 3 Methodological approach.
the impacts of HRC on workers' performance and the indi- vidual factors that affect HRC.
First, a search string was defined when considering three groups of keywords:
-
G1 – Topic: HRC
Keywords: Collaborative robot*; Cobot*; Co‐robot*; Hu- man Robot Collaboration; Human Robot Interaction
-
G2 – Topic: worker and system's performance that might be influenced by HRC
Keywords: Performance; Reliability; Error; Productivity; Efficiency; Safety; Time; Quality; Ergonomics
-
G3 – Topic: The industrial contexts
Keywords: Manufact*; Production; Industr*; Factory
As detailed in [5], all the possible combinations of these keywords (the Boolean ‘AND’ operator between keywords of each group and the ‘OR’ operator within each group), which covered the titles, keywords and abstracts of papers, were used to carry out the search process in the scientific database Sco- pus. The search was limited to articles published in the English language. After this, the articles were selected through a qualitative screening process performed in two different stages. The first stage involved a reading of the titles and abstracts. Papers were included or excluded according to specific exclu- sion criteria, such as papers with no text available or in which HRC was analysed only from a technological point of view, not from the operator's perspective. Instead, the second stage involved reading the full text of the papers selected in the first stage, which allowed us to determine the most relevant ones based on the exclusion criteria described above. Finally, the papers resulting from the second screening were analysed, and for each of them, the main characteristics, methodological approaches and worker's performance as affected by HRC were collected. Furthermore, all the relevant individual factors involved in HRC were examined and classified, allowing us to
define a preliminary taxonomy of key factors that could significantly affect the performance of collaborative operators. Step2, instead, focussed on the analysis of HRA methods.
In particular, the main HRA methods were investigated to provide an overview of the HEP evaluation and PSFs consid- ered in the several HRA approaches. Among the different methods, the Simulator for Human Error Probability Analysis (SHERPA) model [7] was identified as the most suitable for deepening the PSF evaluation in detail. The SHERPA model provides a theoretical framework that exploits the advantages of simulation tools and traditional HRA methods to model the human behaviour and predict HEP for a given scenario in every type of working systems. The performed task, the PSFs and the time worked are considered to estimate human reliability. In particular, the PSFs used in this method were identified and deepened in detail. Although many HRA methods have often proposed numerous PSFs, SHERPA attempts to provide reasonable coverage of the influence spectrum of human per- formance including in the minimum number of eight PSFs all the key factors belonging to the main 4 categories (human, system, task and environment) [17].
Once clarified, which are, on one hand, the main factors influenced by HRC implementation in a manufacturing context, and on the other hand, the PSFs used in the SHERPA method and their impact on the HEP evaluation; through Step 3, the PSF taxonomy for HRC was developed. The PSFs of the SHERPA model were analysed to identify if and how the implementation of collaborative robotics can affect them. For each of the PSFs, it was determined whether the impacts of HRC have been investigated by researchers. For the PSFs affected by HRC, the effects were thoroughly analysed to distinguish the cases of ‘No HRC Impact’, ‘Possible HRC Impact’ and ‘HRC Impact’. No changes were made to the PSFs characterised by a ‘possible impact’ caused by the new type of interaction between humans and cobots. For the other ones, different strategies were pursued. In addition, individual factors
obtained as the results of the scoping literature review that were not considered in terms of impact on existing PSFs were added to the taxonomy. In conclusion, the proposed taxonomy extends and modifies SHERPA PSFs to include the new sce- narios provided by the HRC.
-
| RESEARCH STUDY FINDINGS
In this section, the main results of the three steps described in Section 3 are presented and discussed.
-
| Scoping literature review results: individual factors affecting Human–Robot Collaboration
The database search resulted in 921 papers. Only 177 relevant studies were selected after the initial screening step (title and abstract reading), and finally, this sample was reduced to 27 papers after the second screening step (full‐text reading). The selected papers, which covered a time horizon ranging from
2010 to 2021, were classified according to the methodological approaches reported in Table 2.
The selected papers were deeply analysed by considering the worker's performance influenced by HRC to evaluate the impacts of collaborative robotics on them. Three main di- mensions of HRC impact on worker's performance were as follows:
-
Safety [1, 2, 4, 18–26, 28, 29, 31, 32, 37–40]. -
Ergonomics [4, 18, 22, 24, 26, 27, 31, 32, 37, 41]. -
Productivity [22, 23, 30, 37, 38, 40, 41].
The positive impacts of HRC on workers' performance were confirmed by the analysis. However, some negative im- pacts on workers' behaviour and performance related to the introduction of cobots in industrial contexts were also identified.
Most of the selected papers (20 papers) focussed on safety in HRC. Researchers' interest in this critical aspect makes sense because, in collaborative environments, humans must cooperate
with a cobot in hybrid and fenceless work cells, and this can lead to hazardous interactions between the operator and mobile parts of the machine [18]. To avoid any interference between a robot and human, an obstacle or another moving object, safety assurance mechanisms are required and implemented to meet the corresponding industrial standards [19].
Right after safety, ergonomics is affected by HRC. Three main domains of specialisation for ergonomics (i.e., physical, cognitive and organisational) have been identified by the In- ternational Ergonomics Association. Among these, physical and cognitive factors are the most relevant for HRC. Physical ergonomics is concerned with the physical interactions be- tween humans and other elements of a system [18]; this is positively affected by collaborative robotics, which allows for reducing the biomechanical overload supporting workers in heavy and repetitive tasks that can represent a risk factor [42] and for improving postures [24, 41]. Cognitive ergonomics, instead, focusses on mental processes, especially on cognitive functions and psychological/behavioural level interactions. Close collaboration between humans and robots could provide psychological stress to operators, so the aspects of cognitive ergonomics must be included in the design of collaborative work cells [24, 31]. Finally, organisational ergonomics deals with teamwork, participatory design, community ergonomics, cooperative work, communication and the design of working times to achieve a harmonised work system able to ensure both job satisfaction and the commitment of the employees [18]. No results related to organisational ergonomics in collaborative environments were found in the literature.
Finally, only seven papers analysed the effects of HRC on productivity. This is probably because the improvement in productivity has been taken for granted when collaborative robotics are introduced into a manufacturing system. However, productivity can be influenced by the role taken on by the worker [30].
Regarding the impacts of HRC on workers' performance, the main key individual factors influencing the performance of collaborative operators were investigated. The cobots need to interact with workers, and this does not necessarily improve workers' performance. The introduction of HRC and its consequent success or failure depends on various individual factors, which can significantly affect the performance of
TABLE 2 Classification of papers according to the methodological approaches
Number of

Methodological approaches papers References Features
State of the art or review 11 [1, 2, 18–26] General overview of HRC implementation in industrial contexts Development of a model/methodology 9 [27–35] Model or method to implement, for example, the improvement
of collaboration between humans and robots
Interviews or surveys with users or potential users
-
[36–40] Identification of the key factors for the implementation of collaborative
robotics, changes in operator's working conditions and perceived advantages and drawbacks
Development of a framework 2 [4, 41] Developed frameworks to evaluate HRC considering several aspects

of interaction [4] and examine the transition from a manual cell to a collaborative one [41]